Artifical Intelligence ECG Algorithm Technology
Offering the promise of early detection, enhanced interpretation, and the potential for improved patient care.
Product Overview
Today, heart disease represents the most significant disease burden and cost in healthcare and is the leading cause of death. The major challenge is early identification, as heart disease is only generally symptomatic at an advanced stage which, in turn, negatively affects patient health and increases treatment costs.
Artificial Intelligence-Enhanced Electrocardiography (AI-ECG) Algorithms offer the opportunity to transform cardiovascular medicine and revolutionize the detection of heart disease. The electrical signal of the heart is rich in information that cannot be seen by the human eye. AI enables analysis of this hidden data and creation of diagnostic algorithms.
The ECG is one of the most widely-used medical devices in the world. AI-ECG algorithms enable detection of cardiovascular conditions in a wide variety of health settings which would have previously only been possible in a cardiology environment using cardiac imaging equipment, thereby providing a much more effective tool for heart disease screening.
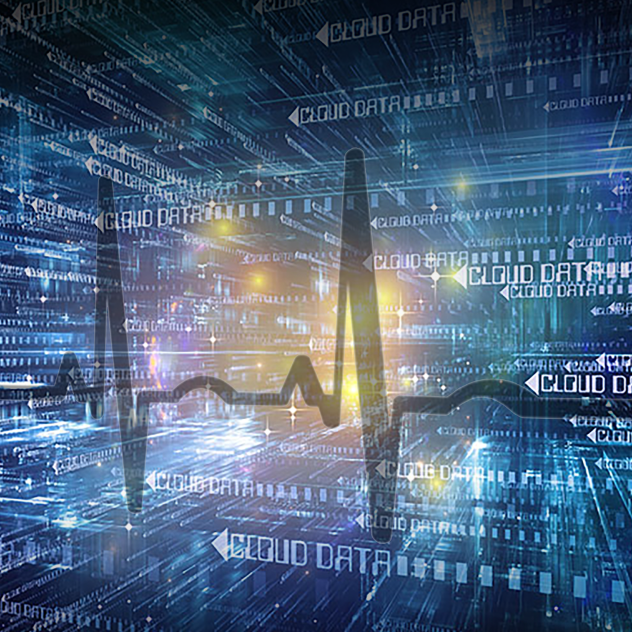
HeartSciences is uniquely developing both cloud and device-based AI-ECG solutions, driving innovation in heart disease detection. This versatility will enable AI-ECG solutions to be provided across a wide range of healthcare settings, from large hospital systems to simple nurse-led mobile testing services.
The MyoVista Insights™ Platform is being designed to provide cloud-based device agnostic AI-ECG algorithms and an AI-ECG algorithm marketplace to host third-party algorithms as well as those developed by HeartSciences.
The MyoVista wavECG™ Device is a high-performance 12-lead resting ECG that combines AI-ECG technology with traditional ECG data, all in one comprehensive test.
AI-ECG Algorithms
HeartSciences has been developing an industry leading portfolio of AI-ECG algorithms using extensive datasets from leading health institutions including the license of significant intellectual property from Icahn School of Medicine at Mount Sinai, New York.
The first AI-ECG algorithm to be incorporated directly into the MyoVista wavECG™ device is impaired cardiac relaxation, which is associated with diastolic dysfunction. HeartSciences has completed patient recruitment for a multi-center clinical study which will be used in the FDA submission.
The first AI-ECG algorithm for submission to the FDA in combination with the MyoVista Insights platform is expected to be Low Ejection Fraction where pre-submission work is currently in progress.
Pre FDA Submission
Left ventricular ejection fraction (LVEF) is the key measure of left ventricular systolic function. Mount Sinai developed a CNN-based AI-ECG algorithm for electrocardiogram (ECG) diagnosis of low left ventricular ejection fraction at <=40%. A total of 715,890 paired ECGs for 147,636 patients were used for training of the algorithm for LVrEF. The model performed extremely well at detecting patients with LVEFs of <=40% both for internal testing (12.52% prevalence) and external validation (25.85% prevalence) with AUROC values of 0.94 (95% CI: 0.94-0.95) in each case. External validation achieved a sensitivity 0.87 (0.85-0.9) with a specificity of 0.85 (0.83-0.87).
Left ventricular ejection fraction (LVEF) is the key measure of left ventricular systolic function. Ejection fraction in a healthy heart is typically in the range of 50% to 70%. Mount Sinai developed a CNN-based AI-ECG algorithm for electrocardiogram (ECG) diagnosis for normal left ventricular ejection fraction at >50%. Results for detection of LVEF>50%. For internal testing (76.7% prevalence), the model achieved an AUROC of 0.89 (95% CI: 0.89-0.89), which was maintained for external validation (59.3% prevalence) at 0.87 (95% CI: 0.87-0.88).
A normal heart will relax quickly during diastole and a stiff heart will move slower. During relaxation, early diastolic filling of the left ventricle occurs at too slow a rate, impairing proper filling of the left ventricle. Left ventricular (LV) diastolic dysfunction is recognized as playing a major role in the pathophysiology of heart failure, however, clinical tools for identifying diastolic dysfunction before echocardiography remain imprecise. A HeartSciences sponsored study at West Virginia University sought to develop AI-ECG models that estimate myocardial relaxation using clinical and electrocardiography (ECG) variables as a first step in the detection of LV diastolic dysfunction. A multicenter prospective study was conducted at 4 institutions in North America enrolling a total of 1,202 subjects. Data from the fourth institution was reserved as an external test set (n=388) to evaluate the model generalizability of the model. The specific echocardiography measurement is called e-prime. Analysis of the AUROC revealed that the estimated eʹ discriminated the guideline-recommended thresholds for abnormal myocardial relaxation and diastolic and systolic dysfunction (LV ejection fraction). The external test sets performed at AUROCs of (0.84, 0.80, and 0.81), respectively. Moreover, the estimated eʹ allowed prediction of LV diastolic dysfunction based on multiple age and sex-adjusted reference limits AUROC of 0.94 in the external sets.
The HeartSciences Impaired Relaxation Algorithm adjusted thresholds are below.
Septal measure < 7.0cm/sec or Lateral measure <10cm/sec for subjects 59 yrs and younger and
Septal measure <6.5cm/sec or Lateral measure <8.5cm/sec for subjects 60yrs and older.
In Development
Mitral Valve Regurgitation is a common valvular condition representing a hidden burden of disease within the population. Valvular disease increases strain upon the heart muscles which can lead to heart failure. The purpose of the study was to develop and validate a CNN-based deep learning algorithm to develop a diagnostic tool to assist in clinical decision making for early detection of moderate-to-severe Mitral Valve regurgitation. For development Mount Sinai used 577,190 ECGs paired to transthoracic echocardiograms from 116,612 patients as training inputs to the model for detection of Mitral Valve Regurgitation. The Area Under Receiver Operating Characteristic curve (AUROC) for the external test set was 0.81 (95% CI:0.80–0.82) using 6860 subjects. Performance was evaluated for the MR classifier with respect to performance in concomitant Aortic Stenosis + MR, and compared this to performance for isolated valvular lesions. It was found that for MR classification, AUROC was higher for patients with concomitant AS + MR using external validation (AS + MR: 0.88; Isolated MR: 0.81).
Aortic stenosis is a common valvular condition that occurs when the aortic valve narrows and blood cannot flow normally. The smaller opening causes your heart's left ventricle to pump harder to push blood through the narrowed aortic valve. Mount Sinai developed a CNN-based deep learning algorithm developed to detect the presence of moderate-to-severe Aortic Stenosis. 585,098 Echo-ECG pairs from 120,564 patients were used for training and 8,111 cohorts for external validation. An AUROC for the external test set achieved 0.86 (95% CI:0.85–0.87). Performance was evaluated for the AS classifier with respect to performance in concomitant AS + Mitral Regurgitation, compared to performance for isolated valvular lesions. We found that for AS classification, AUROC was higher for patients with concomitant AS + MR using external validation (AS + MR: 0.89; Isolated AS: 0.85).
A pulmonary embolism (PE) is life-threatening condition caused by a sudden blockage in your pulmonary arteries, the blood vessels that send blood to your lungs. It may occur when a blood clot in the deep veins of a patient’s leg breaks off and travels to their lungs. A PE is a medical emergency that needs prompt diagnosis and treatment. Current clinical scoring systems for pulmonary embolism (PE) screening have low specificity. Given the current lack of specificity in scoring systems it requires clinicians to use thoracic imaging modalities, most commonly computed tomography pulmonary angiogram (CTPA), to confirm diagnosis. A method for immediate, bedside diagnosis or exclusion of PE is not currently available. A CNN based deep learning algorithm integrating ECG waveforms was developed by Mount Sinai using 23793 CTPAs (10.0% PE-positive), 320746 ECGs and clinical data (demographics, comorbidities, vital signs, and labs) from 21183 patients. A deep learning model AI-ECG model that incorporated limited clinical data performed at an AUROC of 0.81 on the 10% or n=2118 test set. The AI-ECG PE model combined with clinical data may be used to recommend computed tomography pulmonary angiogram or exclude pulmonary embolism in patients with moderate to high likelihood of pulmonary embolism after clinical stratification or those at low suspicion with an abnormal D-dimer. Synergistic deep learning of ECG waveforms with traditional clinical variables can increase the specificity of PE detection in patients with moderate or greater suspicion for PE.
Right ventricular (RV) size and functional metrics have important prognostic implications for many diseases, including cardiomyopathy, pulmonary hypertension, and structural heart disease. Right Ventricle Systolic Dysfunction and Right Ventricle Dilation are not as easily assessed as the left ventricle. Mount Sinai trained a CNN-based AI-ECG model to predict RV dilation (RVEDV >120 mL/m2), RV dysfunction (RVEF ≤40%), and numerical RVEDV and RVEF from an ECG paired with MRI volumetric measurements in the UK Biobank (UKBB; n=42 938). We then fine-tuned the model in a multicenter health system Mount Sinai Hospital n=3019 followed up by prospective validation over 4 months in the same center (MSHvalidation; n=115). The prevalence of RV dysfunction for UKBB/MSHoriginal/MSHvalidation cohorts was 1.0%/18.0%/15.7%, respectively. RV dysfunction model achieved an AUROC performance in the UKBB/ MSHoriginal/MSHvalidation cohorts at 0.86/0.81/0.77, respectively. Deep learning–ECG analysis can identify significant cardiac magnetic resonance imaging RV dysfunction and dilation with good performance. RVEF model AUROC performance was consistent in all tested subgroups, including those with and without LV dysfunction.
Heart failure affects millions globally. The right ventricle plays a crucial role in maintaining overall heart function, and any dysfunction can impact circulation and oxygenation. Right Ventricle Systolic Dysfunction and Right Ventricle Dilation are not as easily assessed as the left ventricle, therefore integrating, and combining multiple parameters may be a more reliable way to determine normal or abnormal function. Development of a CNN-based AI-ECG algorithm was developed by Mount Sinai to analyze ECGs and predict a composite outcome of either RV systolic dysfunction or RV dilation. To develop the algorithm a data set with 761,510 ECGs paired with Echo for 148,227 patients were used. The external test set included 13432 patients for Right Ventricle Dysfunction. The model developed achieved an AUROC of 0.84 (95% CI: 0.84-0.84) during external validation testing. AI-ECG algorithms developed to detect RVD may enhance the usefulness of ECG screening and management for right sided heart failure.
Hypertrophic cardiomyopathy (HCM) is a cardiovascular disease characterized by an abnormally thick heart muscle. Subjects with HCM may not exhibit symptoms but may experience progressive symptoms that can lead to serious heart issues. The HCM algorithm was developed by Mount Sinai using innovative vision-based transformer data science methods. The HCM AI-ECG analysis for diagnosis of hypertrophic cardiomyopathy can aid in early detection of HCM. A total of 78,831 ECGs from four hospitals were used for training and 3,859 ECGs from a holdout set of patients were used for external validation (AUROC: 0.80).
An ST-elevation Myocardial Infarction (STEMI) is a type of severe heart attack caused by an occlusion of one or more of the coronary arteries that supply the heart with blood. A vision transformer-based AI-ECG algorithm was developed to detect STEMI using ECG waveforms that are typically used for detection and diagnosis of STEMI. The STEMI algorithm was developed by Mount Sinai using 21,799 total ECGs for fine-tuning and 4352 ECGs to test the model. The prevalence of STEMI was around 5.4% in the testing set, performance was AUROC: 0.95.
Premature ventricular complexes (PVCs) are prevalent and, although often benign, may lead to PVC-induced cardiomyopathy. Frequent PVCs can lead to changes in the heart’s structure and function causing an irregular heartbeat and/or weakening of the heart muscle. However, PVC induced cardiomyopathy is potentially reversible. A novel CNN-based deep learning ECG algorithm was developed by Mount Sinai using N = 14,241 subjects with 27,370 ECGs in the training set to predict cardiomyopathy in patients with premature ventricular complexes (PVCs). The primary outcome was first diagnosis of LVEF <=40% within 6 months. The model predicted reduction for LVEF <=40% in the external test set (n= 688 and 1,375 ECGs, prevalence 39%) with area under the receiver operating curve of 0.79 (95% CI: 0.77-0.81). This CNN-based algorithm using 12-lead ECG information alone can predict new-onset cardiomyopathy in patients with PVCs, independent of PVC burden, contributing to better patient management.
AI-ECG Cloud Platform
HeartSciences empowers physicians with an efficient and accessible cloud-based tool for ECG analysis using AI algorithms, ultimately benefiting patient care and outcomes. Using cloud platform technology for the diagnosis of ECG results offers several advantages:
Scalability
A cloud platform can handle large volumes of ECG data and seamlessly scale to accommodate.
Accessibility
Access ECG results from anywhere with an internet connection allowing for timely review, and collaboration.
Efficiency
Cloud technology can process ECG data rapidly, providing near-instant results and streamlining the diagnostic process.
Collaboration
Cloud-based solution facilitates collaboration leading to more confident treatment decisions.
Cost-Effectiveness
Avoid significant upfront infrastructure costs and pay only for the resources they use.
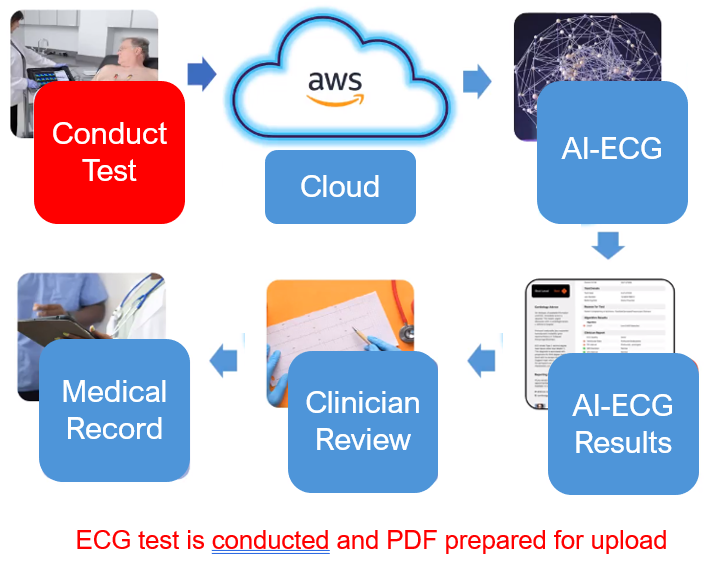
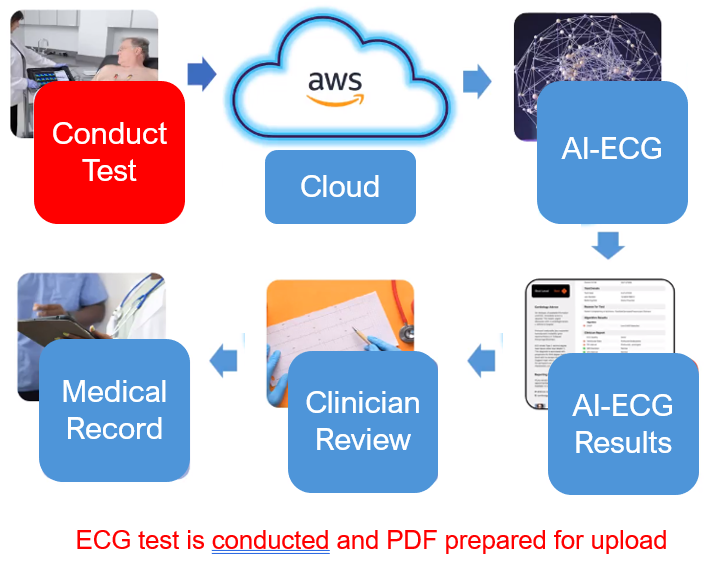
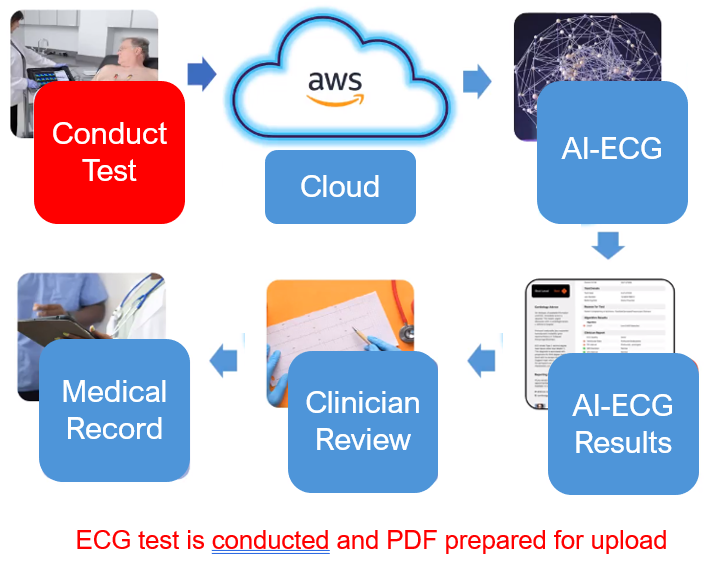
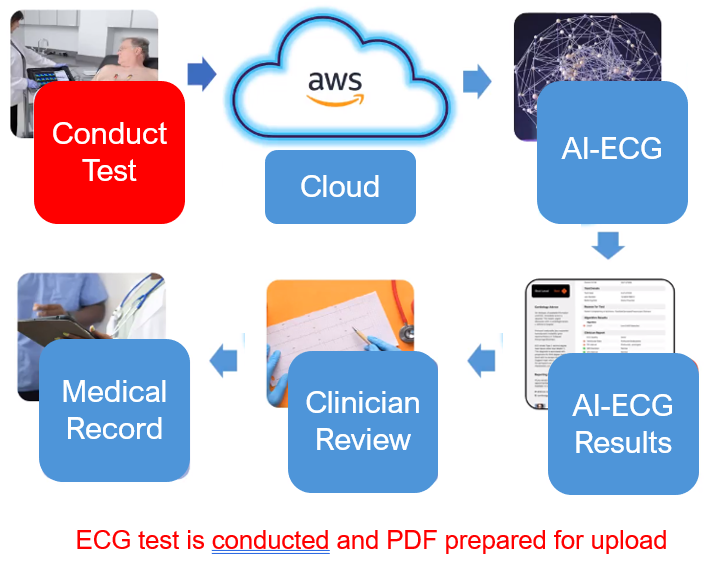
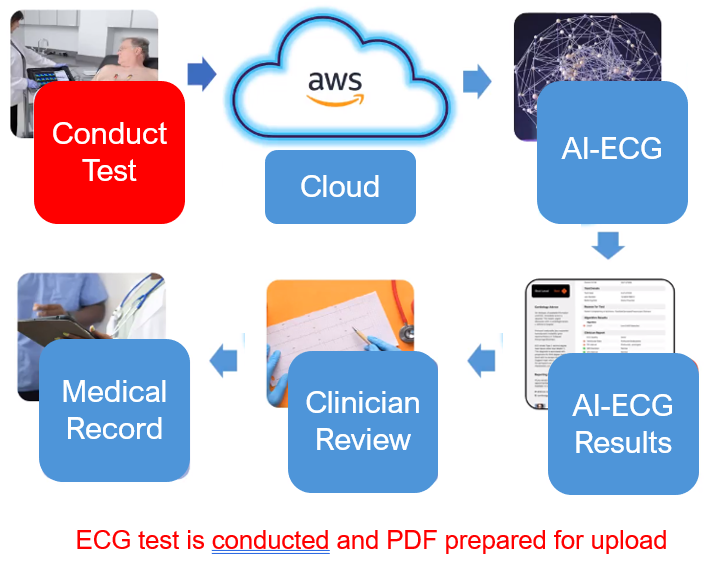
MyoVista Insights™ AI-ECG Cloud Platform
Seamless clinical care and EHR integration.
Delivering AI-ECG algorithms using existing ECG devices across a hospital or health system.
Seamless Integration into Existing Clinical Workflows
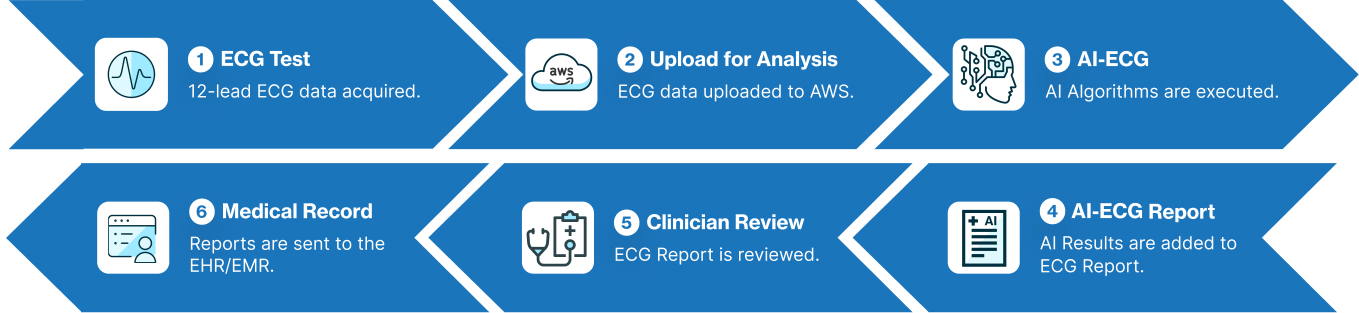
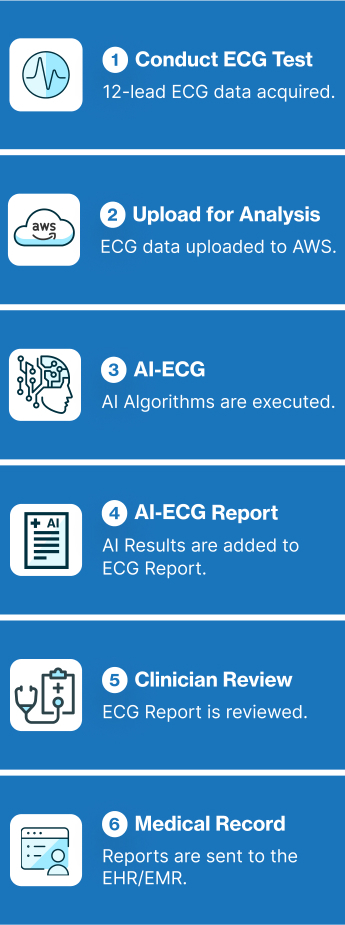
Scalable
No limitation on AI-ECG processing
Accessible
Access AI-ECG anywhere via the internet
Efficient
Instant results
Collaboration
Secure multi-user access to enhance clinical decision making
Cost-Effective
Utilize existing ECG devices. SaaS algorithms mean providers only pay for what is needed
The MyoVista® wavECG™ is a 12-lead resting ECG device designed to provide diagnostic information related to cardiac dysfunction during the diastolic phase* of the cardiac cycle. This diagnostic information has traditionally only been available through the use of cardiac imaging. Now, MyoVista wavECG Technology provides an innovative, in-office advancement for electrocardiographic testing designed to provide physicians with new information to improve patient risk-assessment related to cardiac dysfunction. The MyoVista® Device provides all the information and capabilities of a full-featured conventional resting 12-lead ECG within the same test and follows the same clinical AHA/IEC lead placement protocol.
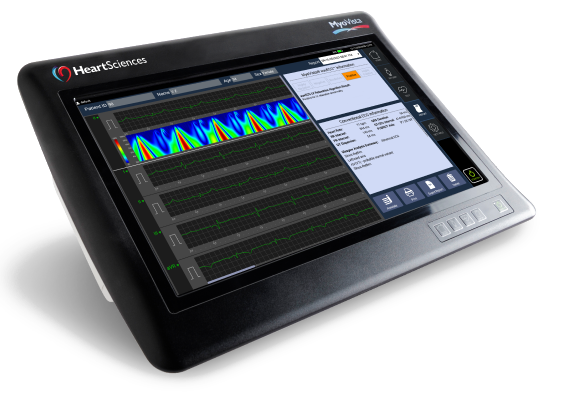
HeartSciences’ Impaired Relaxation AI-ECG Algorithm is a specialized tool designed to analyze electrocardiograms (ECGs) and identify abnormalities by automating the assessment of diastolic function. Extensive research confirms that almost all forms and co-morbidities of heart disease are associated with left ventricular diastolic dysfunction (LVDD) including hypertension, diabetes, valvular disease, ischemia, and reduced systolic function. The MyoVista wavECG AI-based Algorithm is designed to detect LV diastolic abnormalities caused by heart disease and/or age-related cardiac dysfunction.
MyoVista wavECG LV Relaxation Algorithm Result Statements
The MyoVista wavECG LV Relaxation Algorithm is calculated using AI-based algorithms which incorporate data from the transformed ECG signal using continuous wavelet signal processing as well as conventional ECG information. The wavECG LV Relaxation Algorithm Result is provided in both indicator and statement form and displays the result as either “Highly Negative”, “Negative”, “Borderline”, “Positive” or “Highly Positive” for LV relaxation abnormality.
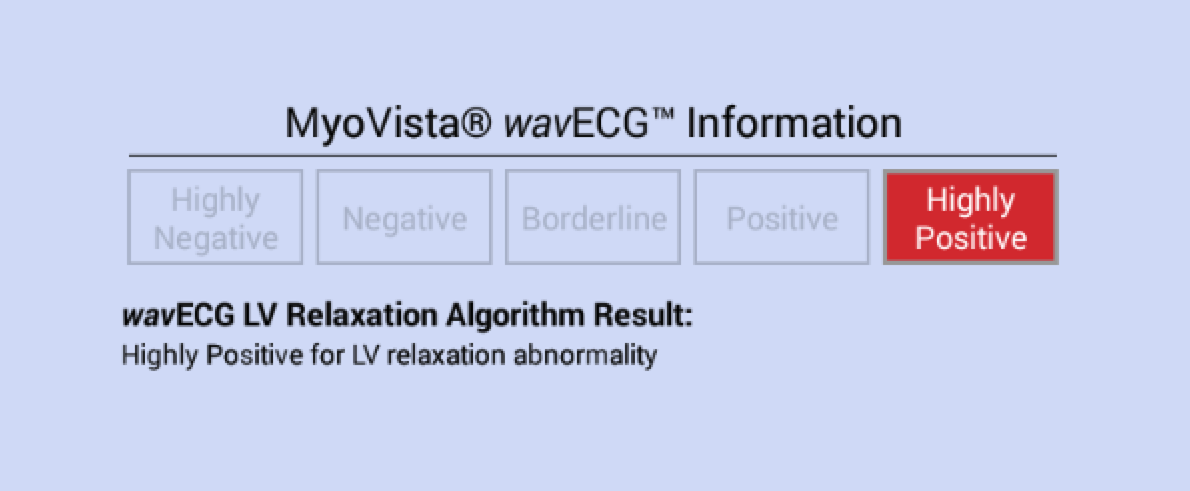
MyoVista® Wavelet ECG (wavECG™):
The MyoVista® wavECG, utilizes an advanced form of signal processing called Continuous Wavelet Transform (CWT).
- CWT extracts valuable frequency information from acquired ECG signals
- The resulting data is then analyzed using artificial intelligence (AI) algorithms.Clinical Impact:
- Undetected heart disease remains a significant challenge in healthcare.
- Millions of ECGs are performed weekly, and HeartSciences aims to revolutionize their clinical utility.
- By combining research, wavelet signal processing, and AI, HeartSciences strives for the early detection of heart disease.
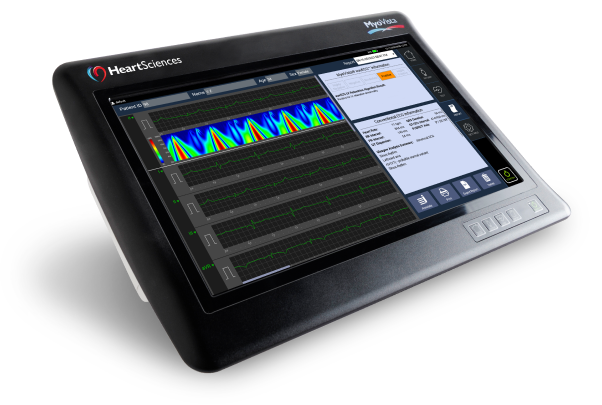
The MyoVista® wavECG™ is a 12-lead resting ECG device designed to provide diagnostic information related to cardiac dysfunction during the diastolic phase* of the cardiac cycle. This diagnostic information has traditionally only been available through the use of cardiac imaging. Now, MyoVista wavECG Technology provides an innovative, in-office advancement for electrocardiographic testing designed to provide physicians with new information to improve patient risk-assessment related to cardiac dysfunction. The MyoVista® Device provides all the information and capabilities of a full-featured conventional resting 12-lead ECG within the same test and follows the same clinical AHA/IEC lead placement protocol.
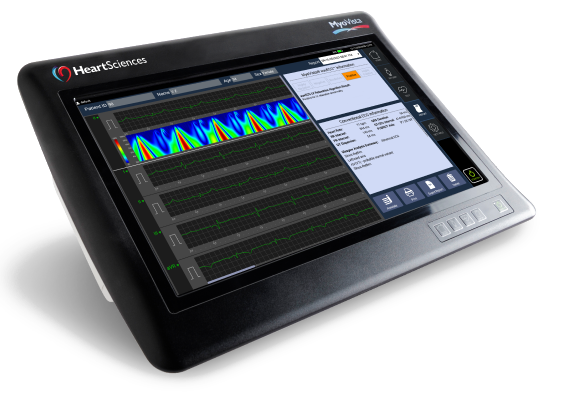
HeartSciences’ Impaired Relaxation AI-ECG Algorithm is a specialized tool designed to analyze electrocardiograms (ECGs) and identify abnormalities by automating the assessment of diastolic function. Extensive research confirms that almost all forms and co-morbidities of heart disease are associated with left ventricular diastolic dysfunction (LVDD) including hypertension, diabetes, valvular disease, ischemia, and reduced systolic function. The MyoVista wavECG AI-based Algorithm is designed to detect LV diastolic abnormalities caused by heart disease and/or age-related cardiac dysfunction.
MyoVista wavECG LV Relaxation Algorithm Result Statements
The MyoVista wavECG LV Relaxation Algorithm is calculated using AI-based algorithms which incorporate data from the transformed ECG signal using continuous wavelet signal processing as well as conventional ECG information. The wavECG LV Relaxation Algorithm Result is provided in both indicator and statement form and displays the result as either “Highly Negative”, “Negative”, “Borderline”, “Positive” or “Highly Positive” for LV relaxation abnormality.
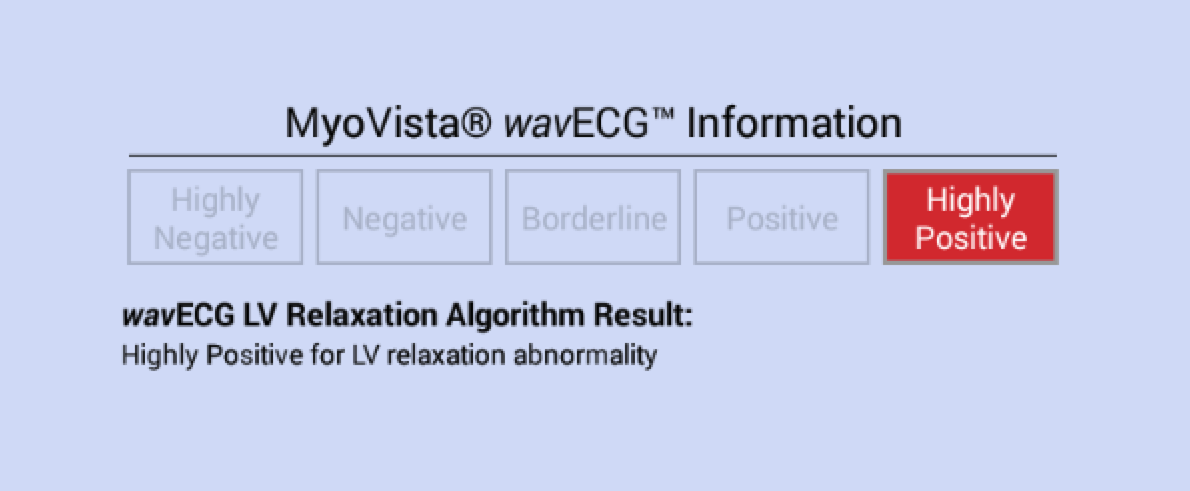
MyoVista® Wavelet ECG (wavECG™):
The MyoVista® wavECG, utilizes an advanced form of signal processing called Continuous Wavelet Transform (CWT).
- CWT extracts valuable frequency information from acquired ECG signals
- The resulting data is then analyzed using artificial intelligence (AI) algorithms.Clinical Impact:
- Undetected heart disease remains a significant challenge in healthcare.
- Millions of ECGs are performed weekly, and HeartSciences aims to revolutionize their clinical utility.
- By combining research, wavelet signal processing, and AI, HeartSciences strives for the early detection of heart disease.
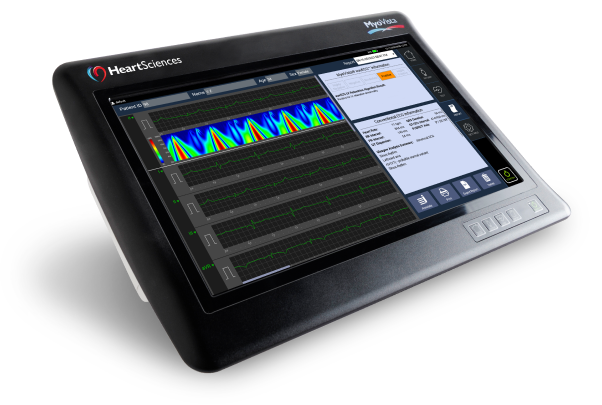
- Provides information related to patient risk for LV relaxation abnormality associated with LVDD
- Displays test result as Highly Negative, Negative, Borderline, Positive or Highly Positive
- Non-invasive
- Follows existing workflow /clinical pathway
- Follows AHA/IEC lead placement protocols
- Includes conventional 12-lead ECG information and University of Glasgow Interpretive Analysis Report within the same test
- PDF and XML file exportable (HL7 capable)
- Quick: 20/30/60 second test and results
- EMR-EHR capable
- Wi-Fi printer enabled
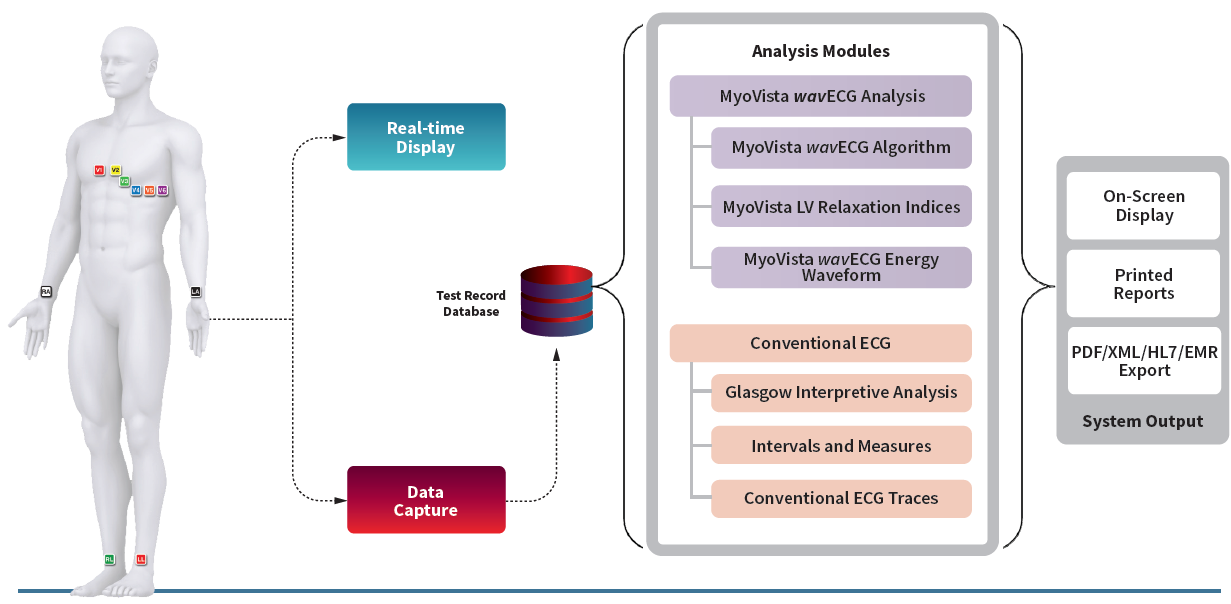
MyoVista® wavECG™ Device
The MyoVista® wavECG™ is a full-featured 12-lead resting ECG with built-in AI-ECG technology. It is portable with a highly customizable, intuitive touch screen user interface. MyoVista® wavECG™ incorporates the AI-ECG impaired cardiac relaxation algorithm and is easily software upgradable to incorporate future AI-ECG algorithms. In the same test, it provides all conventional resting 12-lead ECG diagnostic information including the highly acclaimed Glasgow interpretative analysis and follows usual AHA/IEC lead placement protocols.
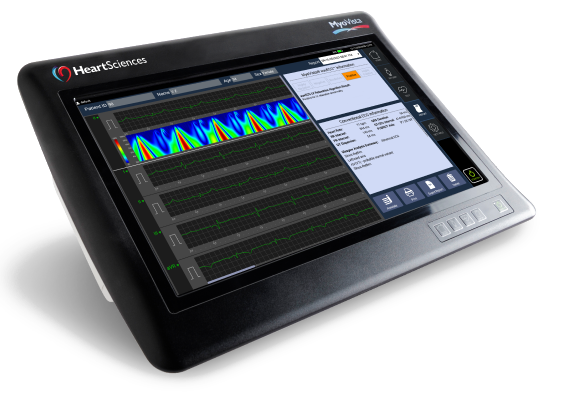
Why an Impaired Cardiac Relaxation Algorithm?
AI-ECG algorithms bring detection of cardiovascular conditions to any healthcare setting, offering capabilities previously limited to specialist cardiology environments using cardiac imaging equipment. This provides a much more effective tool for heart disease detection earlier in the care pathway.
According to the Department of Health and Human Services Public Access: Diastolic Dysfunction and Prognosis 2015:
LV diastolic function [cardiac relaxation phase] is impaired by all of the common pathological processes that affect LV function or produce LV hypertrophy or fibrosis, including hypertension, diabetes mellitus, ischemia, myocarditis, toxins, and infiltrative cardiomyopathies.
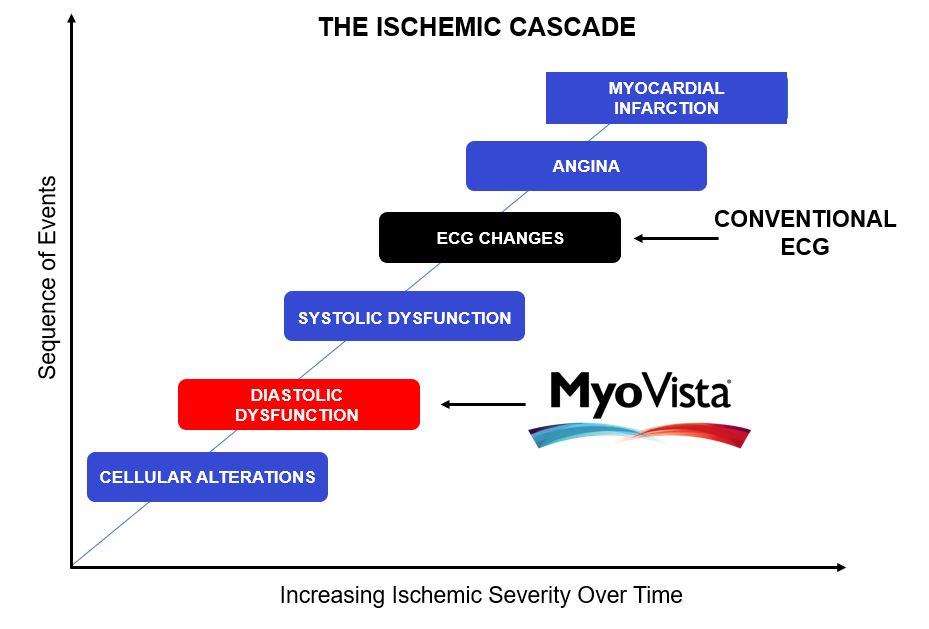
Advanced Signal Processing
The MyoVista® wavECG, utilizes an advanced form of signal processing called Continuous Wavelet Transform (CWT). Valuable proprietary frequency and energy information is extract from the CWT-processed ECG signal.
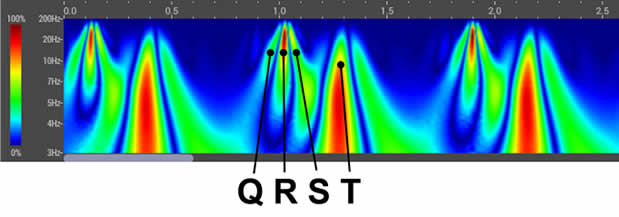
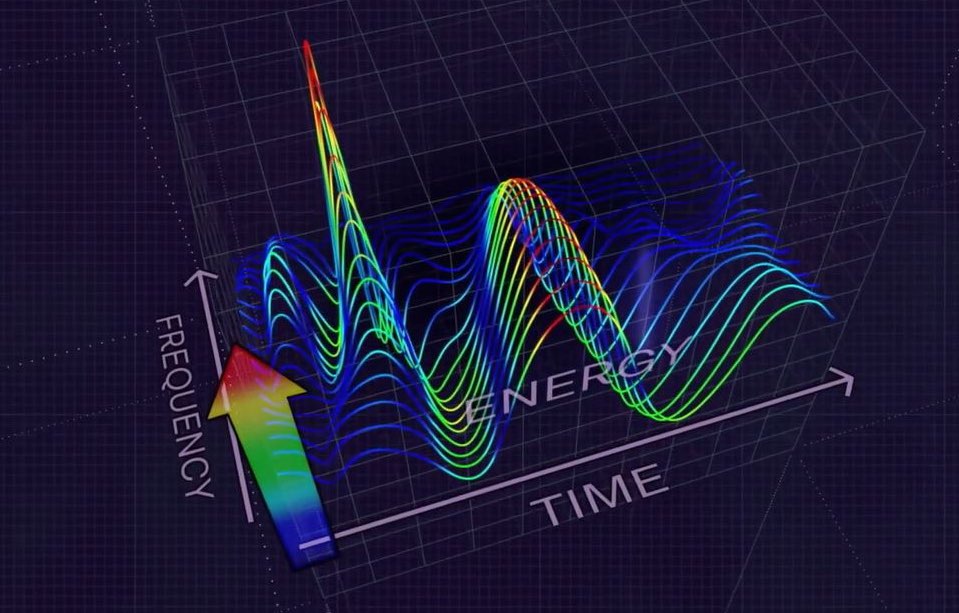